Computer-aided engineering (CAE)
Leverage your CAE data and create surrogate models for performance and fields forecasting
Computer-aided engineering (CAE)
Problems
During design, a great deal of input is required from the designer due to his experience and ability to improve the product.
Design is an iterative process, during which a large amount of data is produced. Most of these will be discarded and forgotten once the final product design is achieved.
Product design based on numerical and experimental simulation generates a large amount of data, in complex formats that are difficult to interpret (individual performances, field quantities, time series, 3D geometries, etc.). Exploring this data to derive useful information for current and future designs is a great challenge.
To develop a new product, it is typically necessary to start from scratch, without exploiting previous know-how, with consequences in terms of time and costs.
Performing complex simulations or preparing experimental setups can be very complex from an economic and time perspective, limiting the number of solutions analyzed in the design and optimization phase.
Making instant and reliable forecasts can greatly speed up product development or the decision chain.
Our Approach
Exploiting your data
Deepyt uses your historical or experimental data from previous datasets and design cycles.
You can export your simulation data (FEA or CFD) and load it directly into Deepyt, using the raw CAD itself or tabular data.
Artificial intelligence algorithms that can be developed with Deepyt make it possible to process all this data and automatically extract a large amount of information, which can be exploited for one’s own purposes.
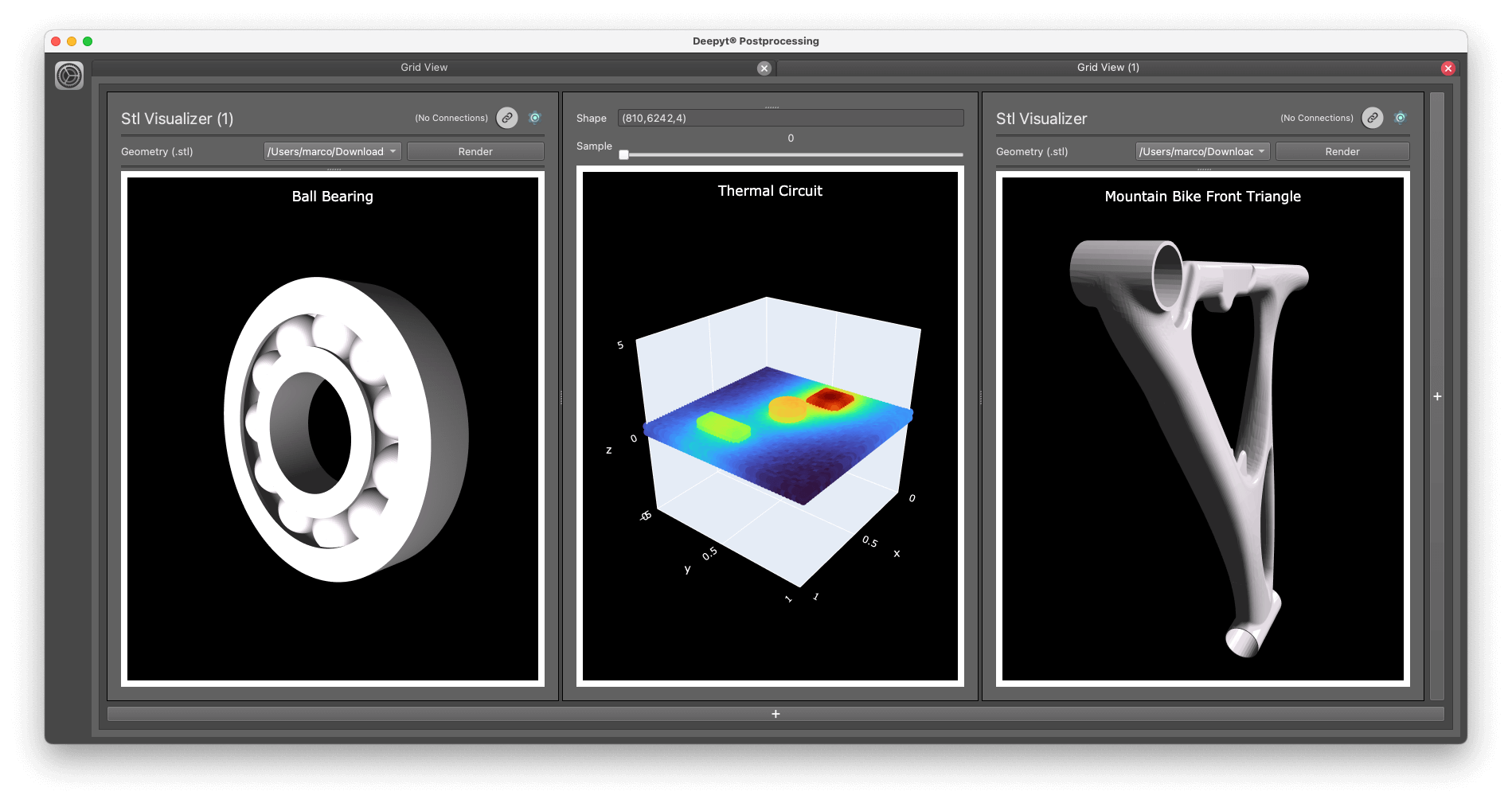
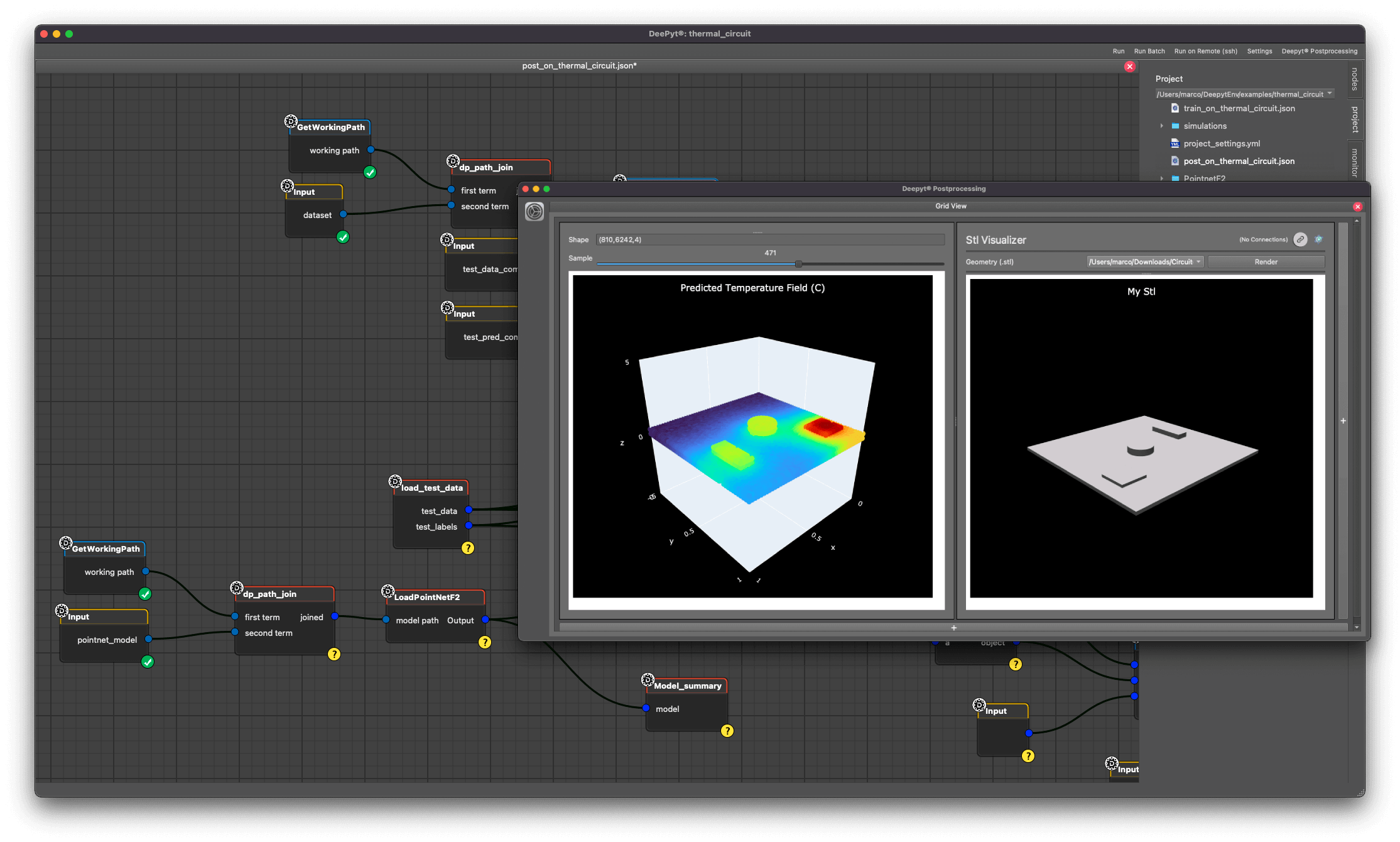
Don't waste knowledge
Using your past projects to develop artificial intelligence algorithms means keeping track of the expertise provided by the designers who contributed to the product design.
Several projects can be analyzed at the same time, extracting all available information, giving value to past experience.
Speeding up design
With our algorithms, it is possible to forecast fluid dynamic/structural performance from pure 3D geometries, tabular data, time series or combinations thereof.
This makes it possible to instantly verify the performance of new designs.
By developing machine learning models and training them on your data you will be able to exploit the metamodel to predict performances, field quantities on surfaces and volumes, time series and so on for new geometries with a very high accuracy, speeding up the design process!
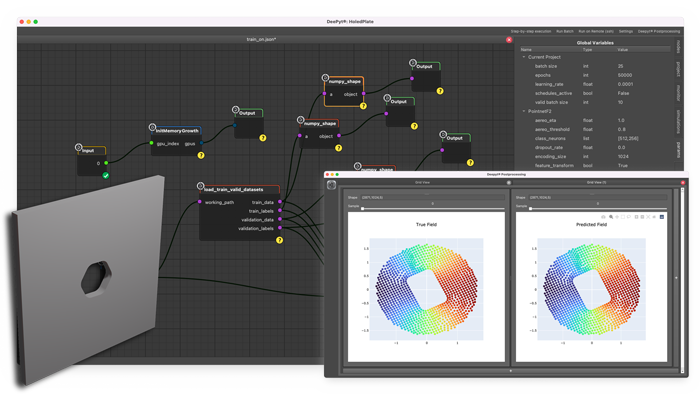
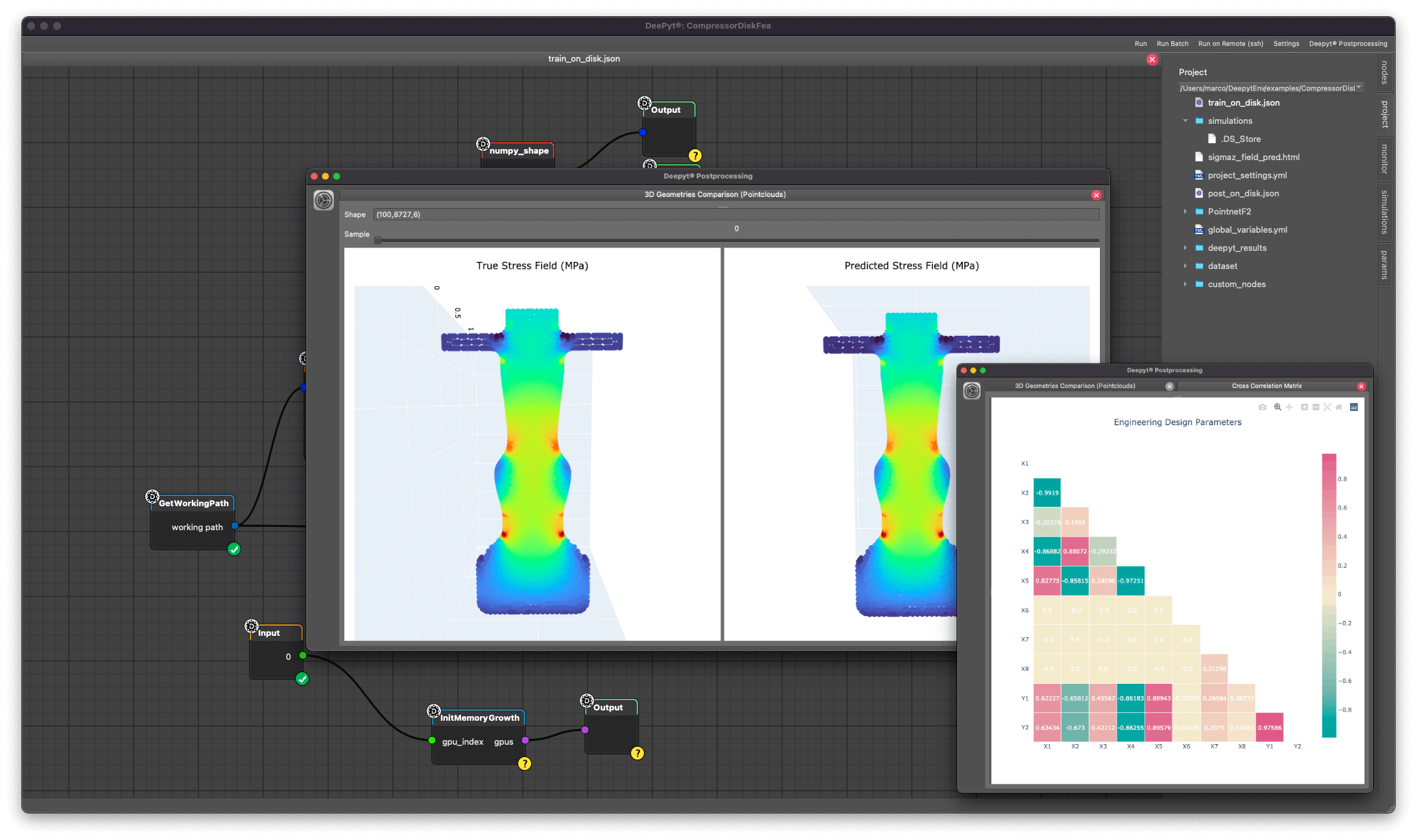
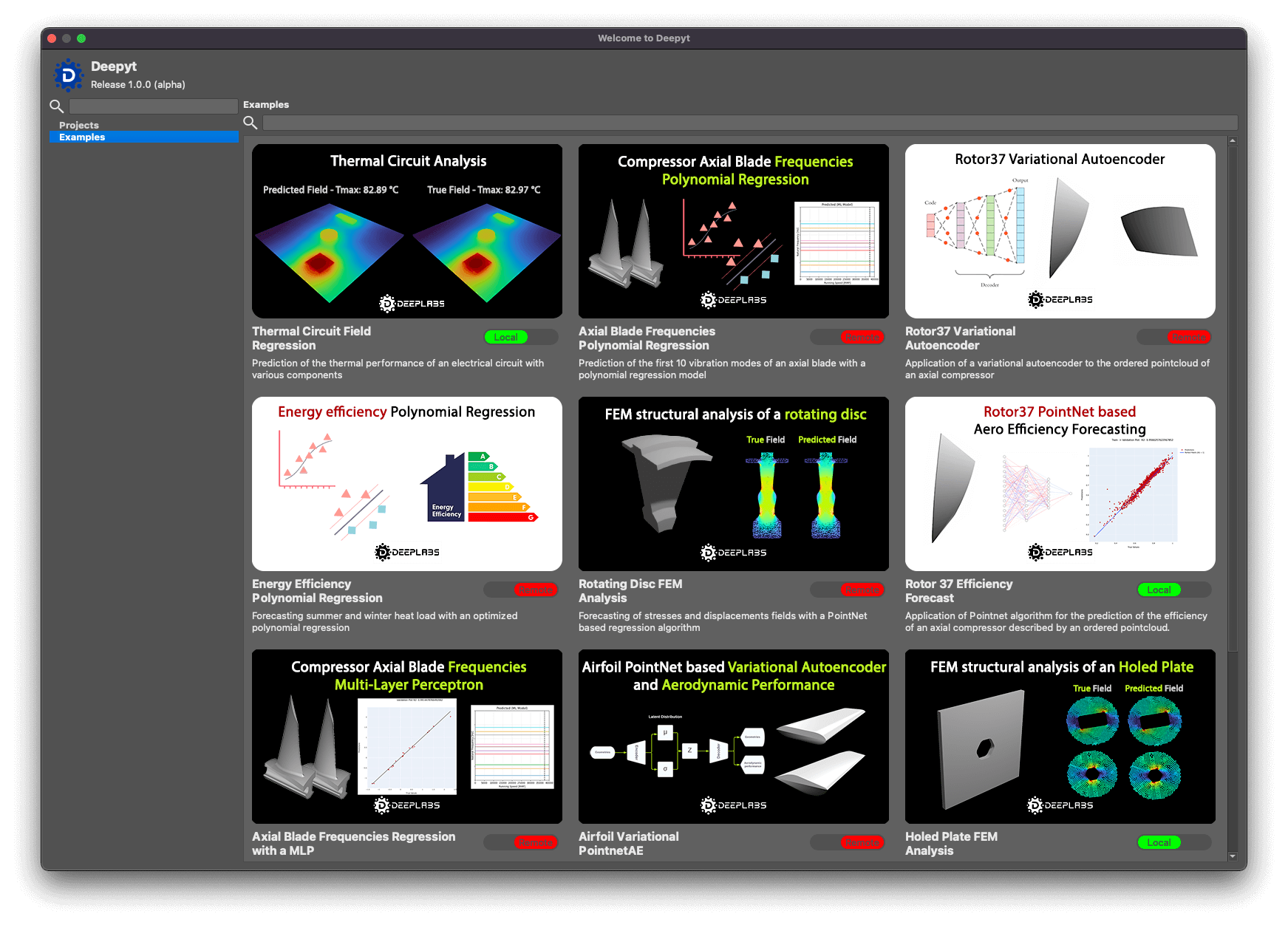